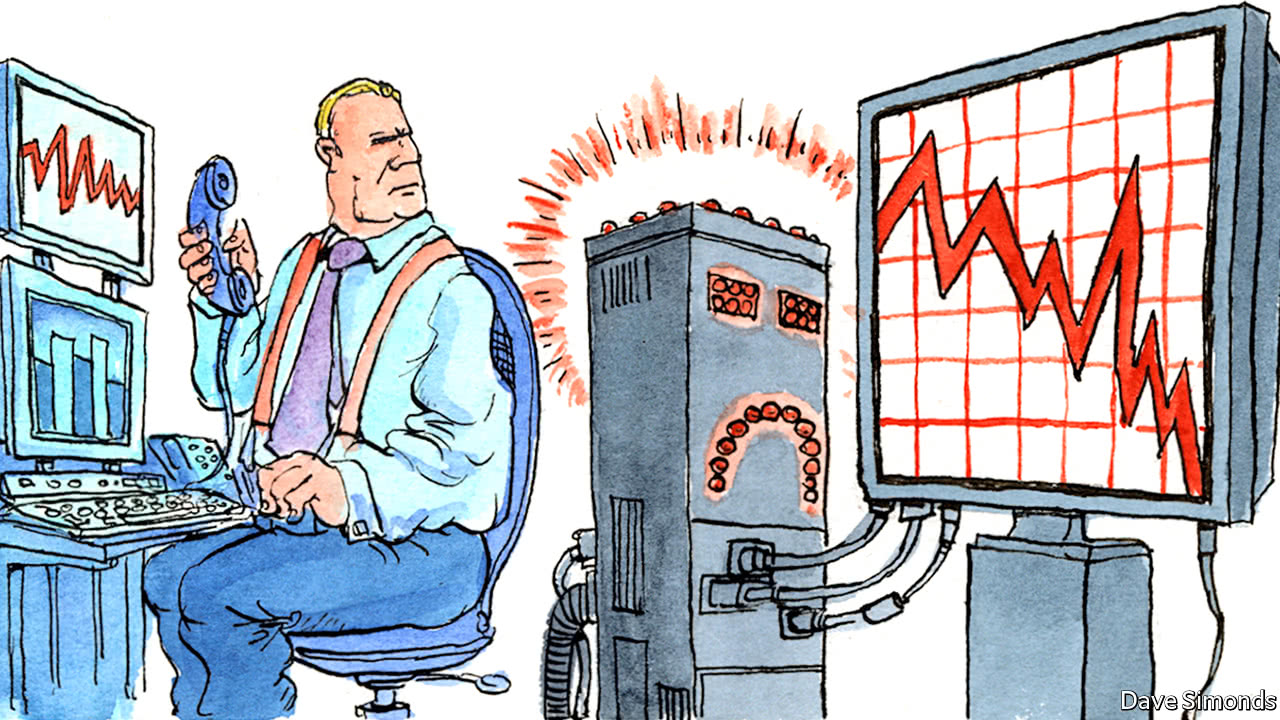
ARTIFICIAL intelligence (AI) has already changed some activities, including parts of finance like fraud prevention, but not yet fund management and stock-picking. That seems odd: machine learning, a subset of AI that excels at finding patterns and making predictions using reams of data, looks like an ideal tool for the business. Yet well-established “quant” hedge funds in London or New York are often sniffy about its potential. In San Francisco, however, where machine learning is so much part of the furniture the term features unexplained on roadside billboards, a cluster of upstart hedge funds has sprung up in order to exploit these techniques.
These new hedgies are modest enough to concede some of their competitors’ points. Babak Hodjat, co-founder of Sentient Technologies, an AI startup with a hedge-fund arm, says that, left to their own devices, machine-learning techniques are prone to “overfit”, ie, to finding peculiar patterns in the specific data they are trained on that do not hold up in the wider world. This is especially true of financial data, he says, because of their comparative paucity. Share-price time series going back decades still contain far less information than, say, the image data used to train Facebook’s facial-recognition algorithms.
-
Putting a price on Bitcoin
-
Theresa May’s Brexit breakfast breakthrough
-
The beginning of the end of China’s “weird architecture”
-
Why did Martin Schulz call for a United States of Europe?
-
Why “death taxes” have fallen out of favour
-
The rise and fall of Al Franken
The trick, then, is to take a more thoughtful approach to deploying AI. Technical prowess obviously matters; Sentient employs a couple of dozen AI experts and constantly researches new methods. But business models matter enormously, too. Sentient started out as a tiny fund a decade ago, managing only its own founders’ money. In the past three years it has expanded into other applications for AI, such as online shopping and website optimisation. Only earlier this year did it launch a hedge fund open to outside money, on which it hopes to apply the insights gleaned elsewhere in its investment arm.
Another San Francisco hedge fund that draws on an even wider pool of expertise, by virtue of its unusual business model, is Numerai, a firm founded in 2015 that launched its first fund this autumn. It starts by taking financial data and then encrypts them so that they are unrecognisable. Its chief operating officer, Matthew Boyd, says this turns them into a “pure math problem”. The idea is that this avoids biases creeping into models—and appeals to Valley types better than the grubby business of picking securities.
It then runs two-stage competitions for machine-learning algorithms that perform best on the data. Some 1,200 data scientists now take part weekly, competing for virtual prizes (in the fund’s own cryptocurrency) in the first round and cash prizes in the second. That structure seeks to encourage algorithms that do well at picking winners over time. The firm takes the results of the best algorithms, decrypts these results back into financial data, and uses the insights to decide which shares to trade. The fund owes at least as much to crowdsourcing, then, as it does to harnessing AI.
One hedge fund that does tout its machine-dependent model, despite naming itself after the human brain, is Cerebellum Capital. Founded as an arbitrage fund in 2008, it started work on a fully AI-run American equity fund in 2016, and launched it in April this year. The fund uses machine learning not just to crunch data and come up with strategies. The classification system that gauges the relative merits of these strategies is itself run by machine learning. But humans do the actual trading, following the algorithm’s instructions.
However they perform in the long term, therefore, one feature of these new AI funds is already clear. At least in investing, more artificial intelligence does not necessarily mean less of the human kind.
Source: economist
Hedge funds embrace machine learning—up to a point